Author: Derek Plemons
It may be a hard pill to swallow, however it’s true: your AI solution is only as good as the data it’s provided. Building a robust analytics foundation is crucial for businesses aiming to extract meaningful insights. Whether you’re a startup or an established enterprise, having a solid analytics framework in place can significantly enhance decision-making processes and drive business growth.
In part 1 of this series, Aligning Data with AI Implementation, we detailed how to get the most value from your AI implementation. Integrating AI capabilities into analytics workflows can unlock new opportunities for predictive modeling, natural language processing, and automated decision-making. From machine learning algorithms to deep learning frameworks, incorporating AI into analytics pipelines can enhance the accuracy and relevance of insights derived from data.
In this article, we’ll explore the essential components of building a strong AI Data Analytics foundation and the key considerations to ensure its long-term effectiveness.
What is AI Data Analytics?
AI Data Analytics refers to the comprehensive approach of using artificial intelligence and machine learning algorithms to analyze large volumes of data, extract meaningful insights, and support decision-making processes. It goes a step further than a simple statistical analysis to include complex pattern recognition, predictive modeling, and autonomous decision-making.

Traditional analytics vs AI Data Analytics
The main difference between traditional analytics and AI data analytics is that the former can be performed without relying on artificial intelligence methods. In traditional analytics, there are two primary approaches: Descriptive and Diagnostic. Descriptive gives you a picture of what happened in the past, while diagnostic answers why something occurred.
Using AI to analyze data can help to process more complex and unstructured data to uncover patterns for making predictions. AI Data Analytics falls into two categories: Predictive and Prescriptive. Predictive analytics tells you what could happen in the future based on past data while prescriptive helps one to determine what should be done to create a certain effect.
Unlike traditional analytics, utilizing advanced artificial intelligence and machine learning systems helps to perform the traditional analytics tasks at a significant increase in efficiency, accuracy, and speed.
The core aspects of AI Data Analytics
1. Advanced analysis: AI can process and analyze complex datasets much faster and more accurately than traditional methods.
2. Pattern recognition: Machine learning algorithms can identify hidden patterns and correlations in data that might be difficult for humans to detect.
3. Predictive analytics: AI can forecast future trends and outcomes based on historical data.
4. Prescriptive Analytics: Aims to guide decision-makers to determine what should be done by providing actionable recommendations.
5. Natural language processing: AI can analyze unstructured data like text from social media, customer reviews, or reports.
6. Real-time analysis: AI systems can process and analyze data streams in real-time, enabling quick decision-making.
7. Continuous Learning: Many AI systems can adapt and improve their analytical models over time as they process more data.
8. Augmented Analytics: AI can assist human analysts by automating data preparation, generating insights, and explaining findings in natural language.
The Benefits:
1. Personalization: AI can tailor insights and recommendations to specific users or scenarios.
2. Scalability: Utilizing AI in your data analytics pipeline can enable your organization to analyze more data at scale.
3. Consistency: Unlike humans, AI systems apply the same criteria consistently across all decisions, reducing bias and variability.
4. Transparency and Explainability: Advanced AI systems can provide explanations for their decisions, which is crucial for accountability and trust.
5. Speed and efficiency: AI can process vast amounts of data and make decisions much faster than humans, allowing for real-time or near-real-time decision-making.
6. Automation: Many data analysis tasks can be automated, reducing human error and increasing efficiency.
Enjoying this insight?
Sign up for our newsletter to receive data-driven insights right to your inbox on a monthly basis.
And the challenges:
When implementing any AI Data Analytics solution there are some challenges that should be taken into consideration.
1. Data Quality and Quantity: Your solution is only as good as the data it’s provided. Having too little or messy data will reduce the model’s predictability.
2. Privacy and Security: Protecting sensitive information while maintaining utility
3. Interpretability: Creating AI models that are transparent enough to lend insight into their decision-making process.
4. Scalability: Ingesting, processing, and managing large datasets efficiently.
5. Integration: Building AI models that integrate with current systems and processes.
6. Ethics: Many ethical concerns should be considered related to privacy, fairness, socioeconomic impact, consent, transparency, and accountability.
7. Skill Gaps: Hiring AI professionals with modeling and domain experience.
8. Cost: Is the return on investment high enough to justify its implementation? Are there simpler solutions that don’t require AI?
9. Model Accuracy and Reliability: As your data changes so may the models that use that data which can cause model drift. Re-training models is necessary so that they perform well on new data.
10. Data Silos: Siloing your data can lead to incomplete insights, data quality, limited AI model training, reduced collaboration, and scalability challenges.
11. Regulatory Compliance: Adhering to the rapidly evolving laws and regulations around AI and data use.
12. Bias: Identifying and mitigating biases in the data which could lead to inaccurate results and unfair advantages.
Tying it all Together
Taking into consideration the key aspects, the benefits, and challenges of AI Data Analytics, there are effective and ineffective strategies for implementing AI in your organization. While implementing AI presents new challenges, RevGen will help design and implement an AI Data Analytics solution catered to your unique needs.
At RevGen Partners, we have helped our clients use AI to create more efficient and insightful analytics applications. If you are interested in harnessing the power of AI for your organization, please reach out to us for a consultation.
Learn more about our AI solutions at our Analytics and Insights page and our AI experience on our Artificial Intelligence page.
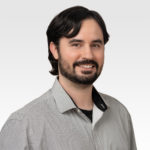
Derek Plemons is a Senior Consultant of Data Science and Artificial Intelligence at Revgen. He has over 5 years of experience leveraging data to solve complex business problems. His knowledge spans various domains that include forecasting, optimization and decision-making algorithms, large language models and cloud computing.